Operationalizing Machine Learning Pipelines for Business Impact

Machine learning (ML) has become a cornerstone of modern business operations, enabling organizations to harness data for insights, predictions, and automation. However, the journey from developing ML models to realizing their full potential in production environments can be complex and challenging. This is where operationalizing ML pipelines comes into play.
5 out of 5
Language | : | English |
File size | : | 1659 KB |
Text-to-Speech | : | Enabled |
Enhanced typesetting | : | Enabled |
Print length | : | 203 pages |
Screen Reader | : | Supported |
What is Operationalizing Machine Learning Pipelines?
Operationalizing ML pipelines involves transforming complex ML models into production-ready systems that seamlessly integrate with existing business processes. It encompasses a series of steps that ensure the efficient and reliable deployment, monitoring, and maintenance of ML models throughout their lifecycle.
Benefits of Operationalizing ML Pipelines
Operationalizing ML pipelines offers numerous benefits, including:
- Improved Model Performance: Continuous monitoring and feedback mechanisms ensure that models remain optimized and perform at their best.
- Increased Business Value: Deploying ML models in production environments directly impacts business outcomes, such as revenue growth, cost reduction, and customer satisfaction.
- Reduced Time to Market: Streamlined operationalization processes accelerate the deployment of ML models, enabling businesses to capitalize on data-driven insights faster.
- Enhanced Collaboration: Operationalizing ML pipelines fosters collaboration between data scientists, engineers, and business stakeholders, ensuring that models align with business objectives.
Key Considerations for Operationalizing ML Pipelines
To successfully operationalize ML pipelines, organizations should consider the following key factors:
- Data Management: Ensure access to clean, reliable data for model training and deployment.
- Model Selection and Evaluation: Evaluate different ML models and select those that best meet business requirements.
- Deployment Infrastructure: Choose the appropriate cloud or on-premises infrastructure for model deployment.
- Monitoring and Maintenance: Establish mechanisms for continuous monitoring, alerting, and maintenance of deployed models.
- Governance and Compliance: Implement policies and procedures to ensure responsible and ethical use of ML models.
Best Practices for Operationalizing ML Pipelines
To achieve successful operationalization, follow these best practices:
- Automate Pipeline Execution: Use tools and frameworks to automate the execution of ML pipelines, reducing manual intervention.
- Use Version Control: Track and manage changes to ML models and pipelines using version control systems.
- Establish Data Pipelines: Develop robust data pipelines to ensure the continuous flow of data for model training and deployment.
- Monitor Model Performance: Implement monitoring mechanisms to track model performance and trigger alerts when necessary.
- Foster Collaboration: Encourage collaboration between data science, engineering, and business teams throughout the operationalization process.
Operationalizing ML pipelines is crucial for unlocking the full potential of data-driven insights. By following best practices and addressing key considerations, organizations can transform complex ML models into production-ready systems that drive tangible business outcomes.
To delve deeper into the intricacies of operationalizing ML pipelines, consider reading the comprehensive book "Operationalizing Machine Learning Pipelines: A Practical Guide to Building and Deploying ML Systems." This invaluable resource provides a systematic approach, case studies, and actionable insights to help organizations navigate the challenges and maximize the benefits of ML operationalization.
5 out of 5
Language | : | English |
File size | : | 1659 KB |
Text-to-Speech | : | Enabled |
Enhanced typesetting | : | Enabled |
Print length | : | 203 pages |
Screen Reader | : | Supported |
Do you want to contribute by writing guest posts on this blog?
Please contact us and send us a resume of previous articles that you have written.
Book
Novel
Page
Chapter
Text
Story
Genre
Reader
Library
Paperback
E-book
Magazine
Newspaper
Paragraph
Sentence
Bookmark
Shelf
Glossary
Bibliography
Foreword
Preface
Synopsis
Annotation
Footnote
Manuscript
Scroll
Codex
Tome
Bestseller
Classics
Library card
Narrative
Biography
Autobiography
Memoir
Reference
Encyclopedia
Michael Mikowski
Chris Dufresne
Robert L Hilliard
Charles Mukhtar
Chloe M Gooden
Cathryn J Prince
Olivia Sagan
Thomas D Wickens
Nils Salzgeber
Cheryl Shea
Charles Hulme
Matthew Wilhelm Kapell
Robert Lau
Chris Lauer
Charles J O Leary
James Kuzner
Christian Seidel
Charles Murray
Darrel Kelly
Chris Adkins
Light bulbAdvertise smarter! Our strategic ad space ensures maximum exposure. Reserve your spot today!
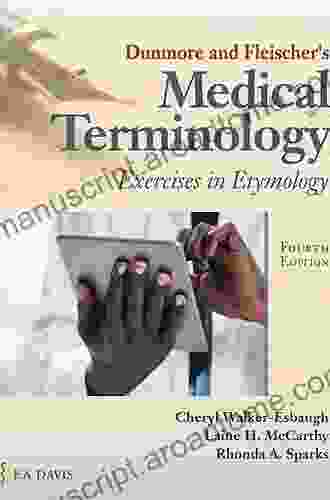

- Dan BrownFollow ·7.8k
- August HayesFollow ·8.2k
- Terry BellFollow ·14.7k
- Guy PowellFollow ·12.2k
- Aubrey BlairFollow ·7.2k
- Herman MitchellFollow ·5.6k
- Robert FrostFollow ·6k
- Jon ReedFollow ·18k
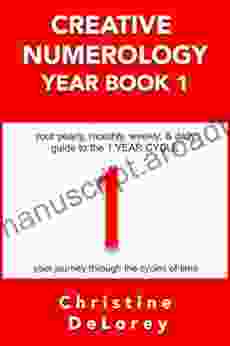

Your Yearly Monthly Weekly Daily Guide To The Year Cycle:...
As we navigate the ever-changing currents...
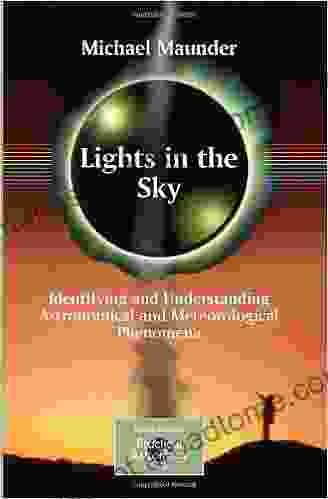

Identifying and Understanding Astronomical and...
Prepare to embark on an extraordinary...
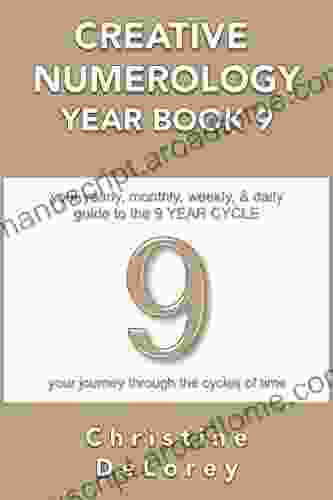

Your Yearly Monthly Weekly Daily Guide to the Year Cycle:...
Welcome to "Your Yearly Monthly Weekly Daily...
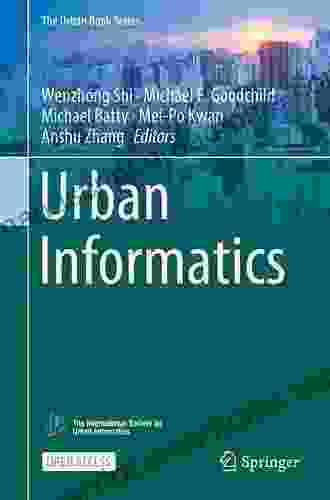

Urban Informatics: Unlocking the Secrets of Smart Cities...
An In-Depth Exploration of Urban...
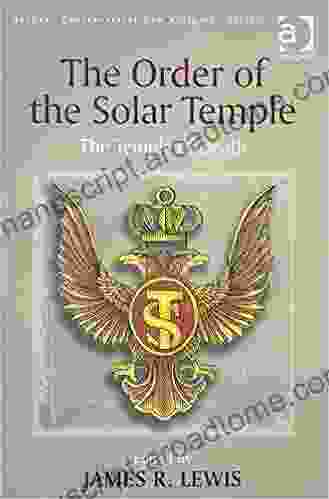

Unveil the Secrets of the Order of the Solar Temple: A...
In the realm of secret...
5 out of 5
Language | : | English |
File size | : | 1659 KB |
Text-to-Speech | : | Enabled |
Enhanced typesetting | : | Enabled |
Print length | : | 203 pages |
Screen Reader | : | Supported |