Unleash the Power of Statistical Inference: Introducing Likelihood Functions

Statistical inference is a fundamental tool for drawing meaningful s from observed data. Among the various techniques employed in statistical inference, the likelihood function stands out as a powerful method that provides a principled approach to parameter estimation and hypothesis testing. This article delves into the concepts and applications of the likelihood function, highlighting its significance and practicality in the field of statistical inference.
The likelihood function, denoted by L(θ; x),is a function of unknown parameters, θ, given observed data, x. It measures the relative plausibility of different parameter values, given the observed data. The likelihood function is proportional to the probability density function (pdf) or probability mass function (pmf) of the observed data, evaluated at the given parameter values.
For a continuous random variable X, the likelihood function is defined as:
4.4 out of 5
Language | : | English |
File size | : | 5270 KB |
Screen Reader | : | Supported |
Print length | : | 348 pages |
L(θ; x) ∝ f(x; θ)
where f(x; θ) is the probability density function of X parameterized by θ.
For a discrete random variable X, the likelihood function is defined as:
L(θ; x) ∝ P(X = x; θ)
where P(X = x; θ) is the probability mass function of X parameterized by θ.
The likelihood function possesses several important properties that make it a valuable tool for inference:
- Maxima: The maximum of the likelihood function corresponds to the most plausible values of the unknown parameters, given the observed data. This property is the basis for maximum likelihood estimation.
- Monotonicity: The likelihood function is monotonically increasing with respect to the probability of the observed data. This means that as the probability of the data increases, the likelihood function also increases.
- Invariance: The likelihood function is invariant under reparametrization. This means that different parameterizations of the same model lead to the same likelihood function.
- Additivity: For independent observations, the likelihood function is the product of the individual likelihood functions. This property is useful for combining information from multiple sources.
The likelihood function serves as a foundation for various statistical inference methods, including:
- Parameter Estimation: Maximum likelihood estimation (MLE) is a method of estimating unknown parameters by finding the values that maximize the likelihood function. This technique yields estimates that are consistent, efficient, and asymptotically normally distributed under certain regularity conditions.
- Hypothesis Testing: The likelihood ratio test is a hypothesis testing procedure that compares two models with different parameters. The likelihood ratio is the ratio of the likelihoods under the null and alternative hypotheses. A large likelihood ratio suggests evidence against the null hypothesis.
- Model Selection: The Akaike Information Criterion (AIC) and Bayesian Information Criterion (BIC) are model selection criteria that penalize models for their complexity. They balance model fit with the number of parameters, aiding in selecting the most parsimonious model.
For a comprehensive exploration of the likelihood function and its applications in statistical inference, I highly recommend the book:
by Gary W. Oehlert
This book provides an accessible and comprehensive to the likelihood function, covering its theoretical foundations, estimation methods, and hypothesis testing procedures. It is an invaluable resource for students, researchers, and professionals in various fields utilizing statistical inference.
The likelihood function is a fundamental tool in statistical inference that enables researchers to draw sound s from observed data. It provides a principled approach to parameter estimation, hypothesis testing, and model selection. By leveraging the likelihood function, statisticians can gain insights into the underlying processes and make informed decisions based on empirical evidence.
4.4 out of 5
Language | : | English |
File size | : | 5270 KB |
Screen Reader | : | Supported |
Print length | : | 348 pages |
Do you want to contribute by writing guest posts on this blog?
Please contact us and send us a resume of previous articles that you have written.
Book
Novel
Page
Chapter
Text
Story
Genre
Reader
Library
Paperback
E-book
Magazine
Newspaper
Paragraph
Sentence
Bookmark
Shelf
Glossary
Bibliography
Foreword
Preface
Synopsis
Annotation
Footnote
Manuscript
Scroll
Codex
Tome
Bestseller
Classics
Library card
Narrative
Biography
Autobiography
Memoir
Reference
Encyclopedia
Chris Minnick
Cecly Ann Mitchell
Tim Woollings
Chris Maxwell
Donald N Yates
Chris Jackson
Christine Daigle
Chris Mcclean
Dad Says Jokes
Leezey Lee
T H G Megson
Charles R Mcconnell
George M Hornberger
Cesar Bravo
Kathryn Warner
Feodor M Borodich
Chris Mcmullen
John M Marston
Charron Monaye
Daniel Pollack
Light bulbAdvertise smarter! Our strategic ad space ensures maximum exposure. Reserve your spot today!
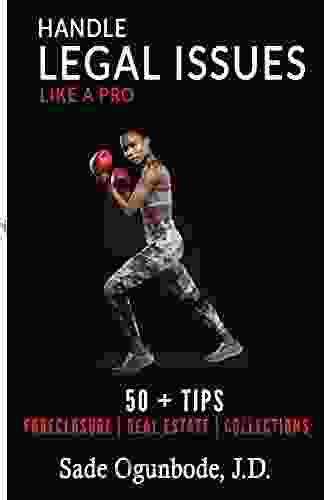

- Hank MitchellFollow ·11k
- Jerome PowellFollow ·2.4k
- Austin FordFollow ·10.6k
- Hugh BellFollow ·7.9k
- Douglas PowellFollow ·10.3k
- John ParkerFollow ·11k
- W. Somerset MaughamFollow ·15.4k
- Rod WardFollow ·14k
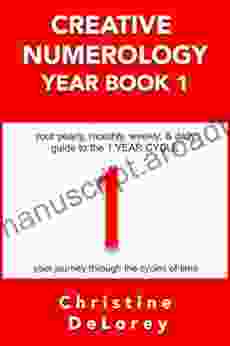

Your Yearly Monthly Weekly Daily Guide To The Year Cycle:...
As we navigate the ever-changing currents...
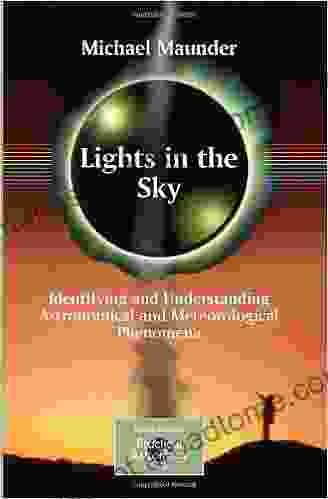

Identifying and Understanding Astronomical and...
Prepare to embark on an extraordinary...
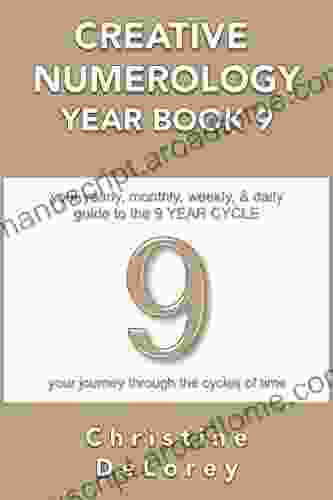

Your Yearly Monthly Weekly Daily Guide to the Year Cycle:...
Welcome to "Your Yearly Monthly Weekly Daily...
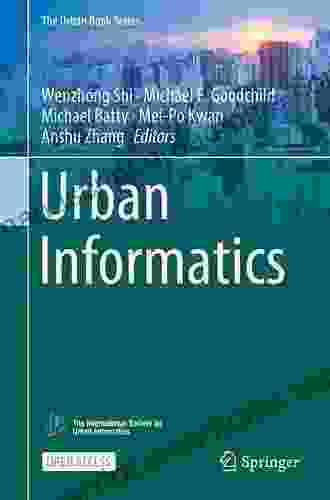

Urban Informatics: Unlocking the Secrets of Smart Cities...
An In-Depth Exploration of Urban...
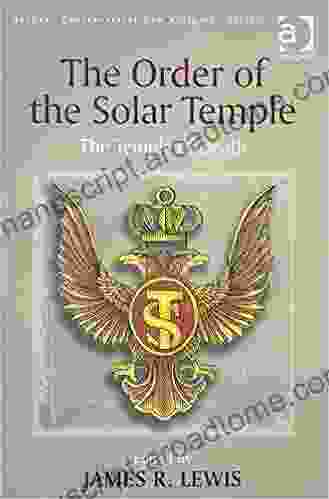

Unveil the Secrets of the Order of the Solar Temple: A...
In the realm of secret...
4.4 out of 5
Language | : | English |
File size | : | 5270 KB |
Screen Reader | : | Supported |
Print length | : | 348 pages |